1 前言#
测试技巧具有普适性,大多是与语言无关的,只是不同语言的生态可能对测试技术的支持各不一样,
比如Python和Java,基本什么库都有,而像C++,有顺手的单元测试和Mock库能用就很不错了。
因为Python比较适合写POC(proof of concept), 而我日常工作的语言是Java+Rust,所以我会穿插着引用这三种语言。
2 Parameterized Test#
在介绍 Parameterized Test 之前,让我们先来看个简单的计算价格与折扣的函数(实际的生产代码肯定会更复杂,但是背后的思路是相通的):
1
2
| def calculate_discount(price, discount_percentage):
return price - (price * discount_percentage / 100)
|
针对这个函数,我们可能会编写多个 test case, 比如价格是 100, 给10%的折扣; 价格是200, 给20%的折扣; 价格是50, 给0的折扣;还有异常case,比如价格为负数的时候,或者折扣为负数的时候.
2.1 单个 test case#
对于这么多的 case, 一个简单粗暴的方式就是把所有的 case 都写在一个 test case 里:
1
2
3
4
5
6
7
8
9
| import pytest
def test_calculate_discount():
# happy path
assert calculate_discount(100, 10) == 90
assert calculate_discount(200, 20) == 160
assert calculate_discount(50, 0) == 50
# unhappy path
# assert calculate_discount(-2, 10)
# assert calculate_discount(10, -2)
|
但是这样的做法一般是不推荐的,Best Practice是一个 test case 只测一种情况,因为如果一个 test case 包含多个测试条件,如果 test case fail 了,那么不看源码或者堆栈,一般还看不出是什么 case 失败了,不好排查。
2.2 多个 test case#
推荐做法就是每个测试条件一个单独的 test case。
另外我们通过test case发现上面的代码没有处理异常情况,我们现在要优化下我们的代码,增加异常处理逻辑(这个就是TDD所推崇的开发哲学, test case 先行,通过test case发现问题,让test case fail掉,然后修正业务逻辑,test case再运行通过).
1
2
3
4
5
6
7
8
9
10
11
12
13
14
15
16
17
18
19
20
21
22
23
24
25
26
27
28
| import pytest
def calculate_discount(price, discount_percentage):
if price < 0:
raise ValueError(f"Price must be greater than zero: {price}")
if discount_percentage < 0:
raise ValueError(f"Discount_percentage must be greater than zero: {discount_percentage}")
return price - (price * discount_percentage / 100)
class TestClassCalculateDiscount:
# happy path
def test_calculate_discount_with_10_discount_percentage(self):
assert calculate_discount(100, 10) == 90
def test_calculate_discount_with_20_discount_percentage(self):
assert calculate_discount(200, 20) == 160
def test_calculate_discount_with_0_discount_percentage(self):
assert calculate_discount(50, 0) == 50
# unhappy path
def test_calculate_discount_with_negative_price(self):
with pytest.raises(ValueError):
assert calculate_discount(-2, 10)
def test_calculate_discount_with_negative_discount(self):
with pytest.raises(ValueError):
assert calculate_discount(10, -2)
|
代码的确是整洁易读了,但话虽如此,我们要多写了很多的 test case.
如果 calculate_discount
变得更复杂,我们要写的 test case 肯定是更多更复杂,总不能都 copy-paste test case吧。
2.3 Parameterized Test#
话题就回到 Parameterized Test 了, 它就是用来解决这个问题的,它可以让你用不同的测试数据集会运行相同的测试逻辑.
还是以上面的代码为例子,你会发现 test_calculate_discount_with_10_discount_percentage
和 test_calculate_discount_with_20_discount_percentage
的测试逻辑是完全一样的,但只是数据集不同,所以我们就可以使用 Parameterized Test 来优化:
1
2
3
4
5
6
7
8
9
10
11
12
13
14
15
16
17
18
19
20
21
| import pytest
class TestClassCalculateDiscount:
# Parameterized test for valid cases (happy path)
@pytest.mark.parametrize("price, discount, expected", [
(100, 10, 90),
(200, 20, 160),
(50, 0, 50)
])
def test_calculate_discount(self, price, discount, expected):
assert calculate_discount(price, discount) == expected
# Parameterized test for invalid cases (unhappy path)
@pytest.mark.parametrize("price, discount", [
(-2, 10), # Invalid price
(10, -2) # Invalid discount percentage
])
def test_calculate_discount_invalid_cases(self, price, discount):
with pytest.raises(ValueError):
calculate_discount(price, discount)
|
其实就是把测试逻辑和数据进行了分离,后面需要测试新的数据集,只需要向数据集里面添加数据即可。
由此可见,使用 Parameterized Test 有几个显而易见的好处:
首先是减少代码冗余,不需要类似的代码 copy-paste 很多次;其次是方便提到测试覆盖率,这个在上面的例子可能不明显,我们可以再修改一下 calculate_discount
函数,增加两个分支:
1
2
3
4
5
6
7
8
9
10
11
| def calculate_discount(price, discount_percentage):
if price < 0:
raise ValueError(f"Price must be greater than zero: {price}")
if discount_percentage < 0:
raise ValueError(f"Discount_percentage must be greater than zero: {discount_percentage}")
if price > 50000:
return price - (price * (discount_percentage * 1.15) / 100)
elif price > 100000:
return price - (price * (discount_percentage * 1.18) / 100)
else:
return price - (price * discount_percentage / 100)
|
价格超过50000, 在已有折扣基础上,再额外给折扣的15%作为折扣;价格超过100000,在已有折扣的基础上,再额外给折扣的18%作为折扣. 如果要覆盖这两个新的分支,只需要在数据集上添加大于50000 和大于100000的数据集,就可以直接覆盖到了.
1
2
3
4
5
6
7
8
9
| @pytest.mark.parametrize("price, discount, expected", [
(100, 10, 90),
(200, 20, 160),
(50, 0, 50),
(50001, 10, 44250.885),
(100001, 10, 88500.885)
])
def test_calculate_discount(self, price, discount, expected):
assert calculate_discount(price, discount) == expected
|
然后测试这段代码的时候,我又发现一个新的问题,这里的价格变成浮点数后,没有作小数点后几位的取整。
(对于这样简单的函数,也能不断地通过写 test case 发现新问题,这无疑就是 test case 最大的价值所在了)
使用 Parameterized Test 还可以提高测试代码的可读性和可维护性,这部分内容还是显而易见的,就不展开了。
2.4 Junit#
在Java的测试生态中,Junit是毫无疑问的龙头大哥,而在Junit5 ,Junit也引入了对 Parameterized Test 的支持,通过 @ParameterizedTest
这个枚举就可以将某个 test case 标注成 Parameterized Test, 通过 @ValueSource
传入待测试数据集:
1
2
3
4
5
6
7
8
9
10
11
| public class Numbers {
public static boolean isOdd(int number) {
return number % 2 != 0;
}
}
@ParameterizedTest
@ValueSource(ints = {1, 3, 5, -3, 15, Integer.MAX_VALUE}) // six numbers
void isOdd_ShouldReturnTrueForOddNumbers(int number) {
assertTrue(Numbers.isOdd(number));
}
|
这只是最基本的用法,Junit还支持通过函数,枚举,CSV格式甚至文件来传入待测试数据集,可谓是包罗万有,具体的用法可以参考这篇文章:Guide to JUnit 5 Parameterized Tests 和 Junit官方文档
2.5 rstest & test_case#
Rust 也有对Parameterized Test支持的库,一个就是 rstest
, 另外一个就是 test_case
, 两者都对 Parameterized Test 有较好的支持,在公司的代码库中,两者我都见过有项目在使用,而我在工作中使用的是 rstest
, 因为它的功能更加强大,维护者也更加活跃.
3 总结#
在了解 Parameterized Test 之前,我的每个CR基本都有 test case 覆盖,但是坐我旁边 Principle Engineer 巨佬 review 我代码的时候,总会说我的 test case 太 verbose 和 heavy, 我在想test case多还不好嘛,我的 code coverage 都超过80%了.
然而他的意思是,不是说我的 test case 没有覆盖到代码,我100行的变更,附上200行的 test case 也没有问题,只不过我的test case大多只是数据不一样,测试逻辑基本相同,能否抽象下,减少下code redundancy, 然后就强烈建议我去看下 Parameterized Test
以及 Property Based Test
.
大佬的确一针见血,我的 test case 大多是复制已有的 test case, 修改下函数名,再加加减减改下数据集。
经他指点,在了解 Parameterized Test
之后,我的确再也没有复制 test case,每次CR的test case也更精简了,CR也更容易通过了.
而他提到的 Property Based Test
则是一项更强大的测试技术,下回再分解了。
系列文章:
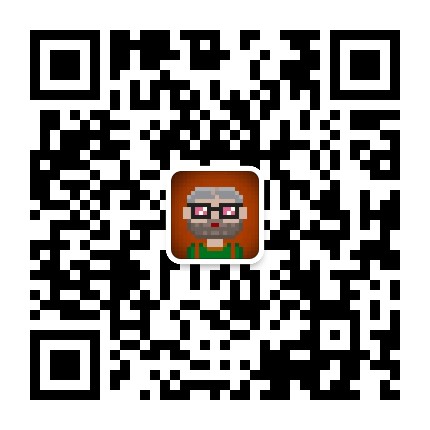
公号同步更新,欢迎关注👻